In This Story
A new study from College of Health and Human Services’ researchers validates the use of artificial intelligence and seasonality to screen patients and identify the probability of COVID-19 prior to testing.
New COVID-19 variants continue to spread and doctors and nurses struggle to differentiate between COVID-19, the flu, and other respiratory diseases before a lab can confirm the results. According to the Centers for Disease Control and Prevention website, “You cannot tell the difference between flu and COVID-19 just by looking at the symptoms alone because they have some of the same symptoms;” however a new study has confirmed computer models can assist with this.
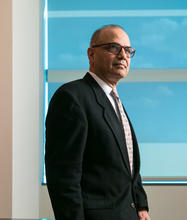
In an earlier study, Professor in the Department of Health Administration and Policy Farrokh Alemi and his research team found that a computer model can predict the probability of COVID-19 vs. the flu based on a small set of Chinese and American data. In a follow-up study that included more scenarios, more symptoms, and U.S.-only data, the team has validated that the constructed computer model can help doctors, nurses, and community health workers diagnose a respiratory illness before laboratory data is available.
“This new study validates the findings from our previous study—our model has the ability to differentiate diseases and provide accurate predictions for diagnoses using only symptom data,” said Alemi. “If built for clinical use, the computer model can assist health care professionals in recommending the correct treatment to a patient who is awaiting lab results.”
Read more about the implications of this and the previous research.
This new study examines differential diagnosis of COVID-19 under 45 different scenarios regarding the spread of COVID-19, influenza, or influenza-like illnesses. Data came from the Centers for Disease Control and Prevention data on laboratory confirmed cases, and the control data came from the Influenza Research Database of the National Institute of Allergy and Infectious Diseases.
The models constructed and validated in this study would not be applicable to asymptomatic individuals or those without respiratory symptoms. Additionally, this study was conducted before the delta or omicron variants of the novel coronavirus emerged, and more data would be needed to establish the accuracy of the model across all variants.
“Differential Diagnosis of COVID-19 and Influenza” was published in PLOS Global Public Health in July 2022. The Mason research team included Associate Professor of Health Informatics Janusz Wojtusiak, Professor of Global Health and Epidemiology Health Amira Roess, Affiliate Faculty Jee Vang, and doctoral student Elina Guralnik, along with Rachele Peterson and Praduman Jain from Vibrent Health. This study was presented at the AcademyHealth Annual Research Meeting in June 2021.
This research was funded by the National Cancer Institute (NCI) to Vibrent Health, Inc. and conducted in collaboration between Mason and Vibrent teams. The study is one of a series of studies that the Mason-Vibrent team conducted to elucidate the utility of symptom-screening algorithms for accurate diagnosis of COVID-19.